blog post
A New Sleigh for Santa from MIT Labs
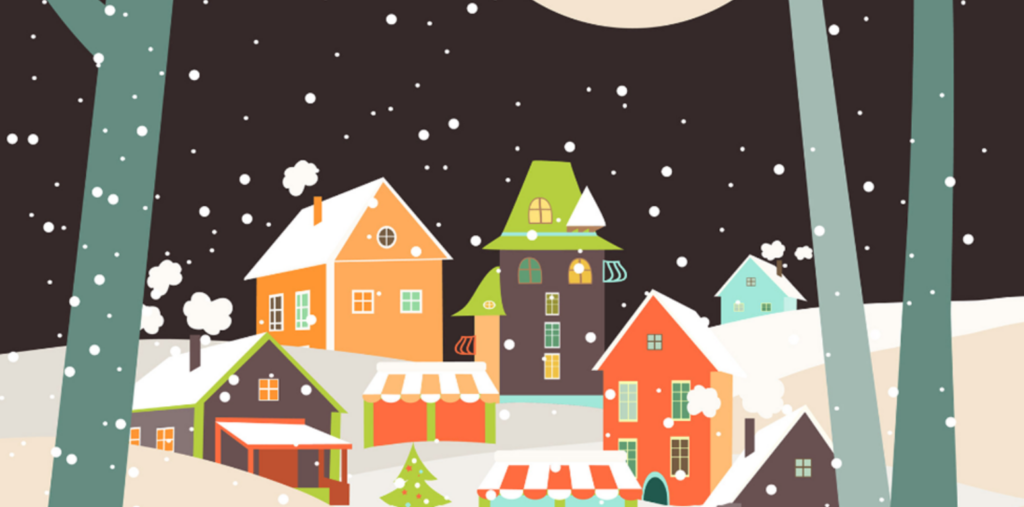
A breakthrough from MIT and ETH Zurich researchers is set to revolutionize how we tackle some of the most head-scratching optimization problems – from the routing of global packages to the operation of power grids. Think of it as a radical upgrade from Santa Claus’s magical sleigh to a data-driven, super-efficient delivery system.
At the center of this cutting-edge development is a machine-learning technique that promises to significantly accelerate mixed-integer linear programming (MILP) solvers. These solvers, often used by companies like FedEx, face Herculean tasks in optimizing complex problems. They typically break down a massive challenge into bite-sized pieces, employing algorithms to unearth the best solutions. But the process is so time-consuming that often, companies have to settle for less-than-ideal solutions due to time constraints.
Genius Solution
This is where the genius of these researchers comes into play. They’ve identified a critical bottleneck in the MILP process – a step so laden with potential solutions that it slows down everything. By employing a filtering technique to simplify this step and integrating machine learning, they’ve managed to find optimal solutions for specific types of problems far more rapidly.
The beauty of their method lies in its tailor-made approach. It enables a company to use its data to customize a general-purpose MILP solver for its specific problem. The results are nothing short of spectacular – speeding up MILP solvers by 30 to 70 percent without sacrificing accuracy.
Imagine the implications of this approach. It can be applied anywhere MILP solvers are used, be it in ride-hailing services, electric grid operations, or vaccine distribution. It’s a game-changer for any entity grappling with complex resource-allocation problems.
Senior author Cathy Wu, an AI trailblazer, and her team are demonstrating a beautiful synergy between machine learning and classical solutions. Wu’s vision is clear – it’s not about choosing between machine learning and traditional methods but harnessing the best of both worlds for groundbreaking results.
ML to the Rescue
MILP problems are notoriously challenging, with potential solutions often outnumbering the atoms in the universe. Wu and her team’s innovative approach simplifies this immense solution space, using machine learning to choose the most effective combination of algorithms from a significantly reduced pool of options.
This isn’t just about efficiency; it’s about precision and adaptability. The machine-learning model is trained on data specific to the user’s optimization problem, making it increasingly adept at tackling tasks tailored to the user’s needs. This iterative learning process is a form of reinforcement learning, constantly evolving to find better solutions.
Applied Science
Looking ahead, Wu and her team are aiming to apply this groundbreaking approach to even more complex MILP problems, potentially transforming how we tackle some of the most intricate challenges in logistics, energy management, and beyond. And it is in those areas, particularly energy management and the electrical grid, air travel and healthcare to name just a few top prospects that need help the most.
Today, a handful (like 7 at last count) of minimally folks from TSA oversee the most complex energy grid on the planet and cannot predict or prevent any style of cyber-disaster from happening. Why? I don’t know.
Medical centers all over the country now allocate over 25% of a doctor’s time to “paperwork” and most practitioners consider the prevention of cyber-attacks as not what they spent 12 years in medical school learning how to do.
If a large transformer in one of our sub-grids is crippled by a cyber-attack, we have no plan B. They are big, built from a customized template, virtually impossible to replace because we have no backups and most are made in Europe. Just getting them to their location would be challenging.
Flying airplanes still requires us to removed belts, shoes and jewelry, even though a future attacker would never use a shoe bomb as an alternate to a cyber-attack, yet fully 25% of the DHS are TSA employees.
We better stop screwing around and start taking all of this seriously yesterday, or that next attack and explosion will surprise everyone.
Author
Steve King
Managing Director, CyberEd
King, an experienced cybersecurity professional, has served in senior leadership roles in technology development for the past 20 years. He has founded nine startups, including Endymion Systems and seeCommerce. He has held leadership roles in marketing and product development, operating as CEO, CTO and CISO for several startups, including Netswitch Technology Management. He also served as CIO for Memorex and was the co-founder of the Cambridge Systems Group.