blog post
GPT Architecture
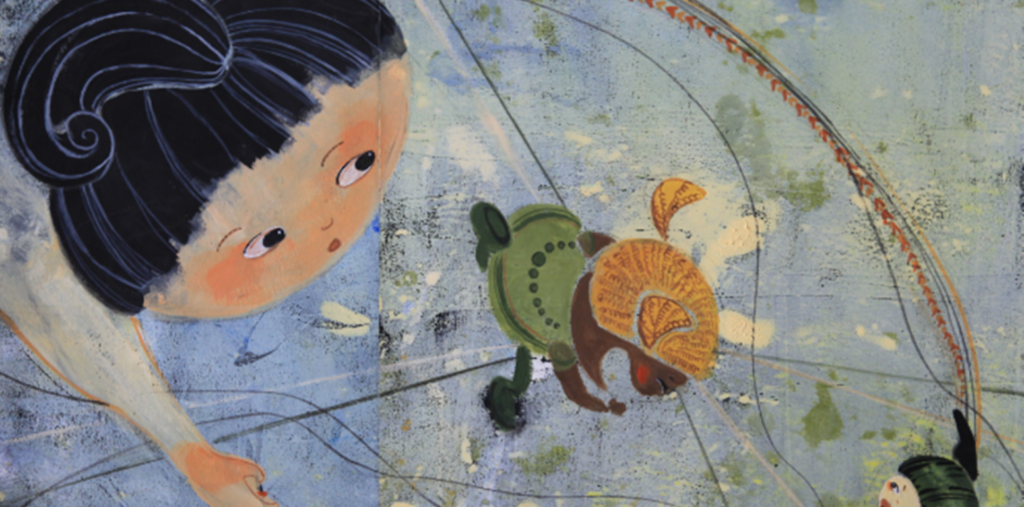
Humans can swiftly grasp a new idea and apply it in various contexts. For instance, once children grasp the action “skip,” they intuitively know what “skip around the room twice” or “skip with raised hands” means.
But can machines emulate this cognitive ability? In the late 1980s, thinkers Jerry Fodor and Zenon Pylyshyn argued that artificial neural networks, the backbone of AI and machine learning, couldn’t make such contextual connections, termed “compositional generalizations.” The subsequent years saw efforts to endow neural networks with this skill, leading to mixed results and continuing the debate.
A collaboration between New York University and Spain’s Pompeu Fabra University has birthed a method, as discussed in the journal Nature, that enhances tools like ChatGPT to execute compositional generalizations. Named Meta-learning for Compositionality (MLC), this method not only matches but at times surpasses human abilities. Unlike previous systems that anticipated compositional generalization from standard training or relied on special architectures, MLC emphasizes honing these skills through focused practice.
Brenden Lake from NYU states, “For over three decades, the capacity of neural networks to achieve human-like systematic generalization was a topic of contention among scholars from diverse fields. Our findings indicate that a regular neural network can match or even surpass human systematic generalization.”
To amplify compositional learning in neural networks, the team developed MLC. It’s an innovative learning approach wherein a neural network constantly evolves its abilities across a range of episodes. In one episode, MLC might learn a term like “jump” and subsequently generate combinations like “jump twice” or “jump right two times.” With each episode featuring a new term, the network refines its compositional proficiency.
To validate MLC’s effectiveness, Lake and Marco Baroni from Pompeu Fabra University designed human-involved experiments identical to MLC’s tasks. Participants didn’t just learn real words but were also introduced to fictional terms like “zup” and “dax”, which they had to contextually apply. Remarkably, MLC’s performance rivaled and sometimes even exceeded human performance, outdoing even advanced models like ChatGPT and GPT-4 in this specific task.
Baroni notes, “Despite their advancements, models like ChatGPT still face challenges with compositional generalization. We believe MLC offers a potential avenue to refine these models further.”
ChatGPT and other models based on the GPT architecture primarily rely on patterns learned from vast amounts of text data to respond to user prompts. They don’t inherently “solve” the challenge of compositional generalization in the same way that a dedicated method like Meta-learning for Compositionality (MLC) might. Instead, GPT-based models predict the next word in a sequence based on patterns they’ve observed in their training data.
However, the strength of models like ChatGPT lies in their ability to generalize from the patterns they’ve seen, even if they aren’t specifically tailored for compositional tasks. If, during their training, they’ve been exposed to a lot of examples that demonstrate compositional reasoning, they might be able to perform it to some extent. However, they might still struggle with certain compositional tasks compared to methods explicitly designed for it, like MLC.
Over time, as the field of AI research advances, techniques and methods will likely be developed or integrated into models like ChatGPT to enhance their abilities in specific areas, including compositional generalization. But while the exact mechanisms and methods would depend on the advancements and findings in the research community, you can bet on one thing and that is the inevitability of integral MLC sometime soon.
Author
Steve King
Managing Director, CyberEd
King, an experienced cybersecurity professional, has served in senior leadership roles in technology development for the past 20 years. He has founded nine startups, including Endymion Systems and seeCommerce. He has held leadership roles in marketing and product development, operating as CEO, CTO and CISO for several startups, including Netswitch Technology Management. He also served as CIO for Memorex and was the co-founder of the Cambridge Systems Group.