blog post
How Generative AI Works
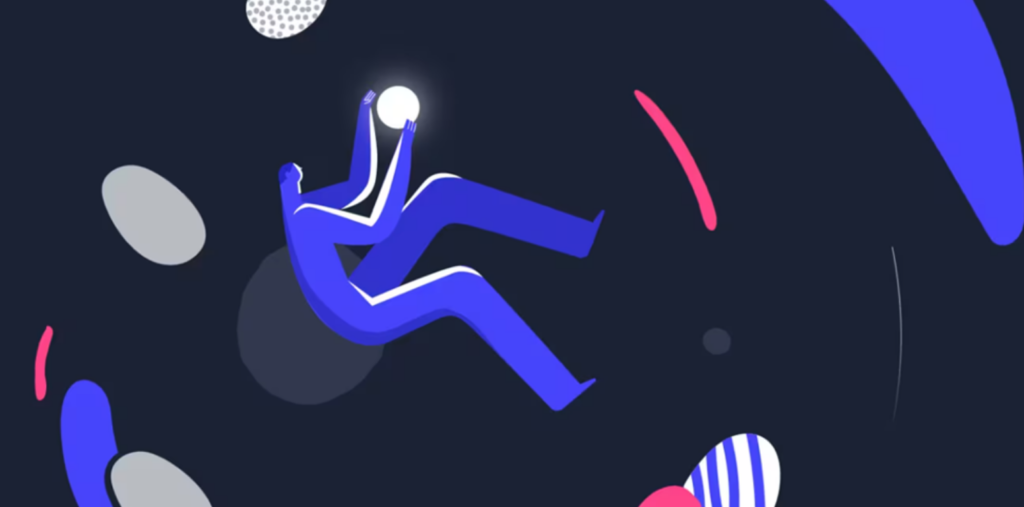
Introducing Charlotte
Generative AI is a branch of artificial intelligence that focuses on generating new data based on existing data. This sophisticated technology enables a variety of use cases — such as data retrieval and analysis, content generation, and summarization — across a growing number of applications.
Generative AI has many applications within the context of cybersecurity, from assisting threat hunters with data retrieval for ongoing investigations to providing real-time insights that inform vulnerability management workflows.
How generative AI works: a brief overview
Generative AI comes out of a subset of AI known as machine learning (ML). ML involves the use of algorithms that improve automatically by learning patterns from vast amounts of data. Among the various domains of ML is deep learning, which uses layered algorithms (called neural networks) that mimic the way neurons function in the human brain. This enables systems to learn and make decisions autonomously.
Within deep learning, we have a type of design for a neural network called a transformer. The transformer model uses layers of artificial neurons that analyze input data in parallel, making the process incredibly efficient. One of the most well-known of these models is the Generative Pre-Trained Transformer model, commonly known as GPT. Pre-trained on large amounts of data, these models can generate eerily human-like text.
Put simply, generative AI involves the following steps:
- The model begins training on a massively large dataset
- The model learns and understands the underlying patterns and structures in the data
- The generative process enables the creation of new data that mimics these learned patterns and structures
With this understanding in place, let’s shift our attention to consider the role that generative AI has in the realm of cybersecurity.
Generative AI in cybersecurity
The potential for generative AI to impact the cybersecurity space is tremendous. Just as it can learn and replicate patterns in text, it can also learn from patterns found in cyber threats or vulnerabilities, or learn the documentation of security products to enable analysts to rapidly query their security tools.
A generative AI model trained on vast amounts of historical cybersecurity data could identify patterns and trends, resulting in the ability to predict future threats. Rather than responding to threats as they occur, cybersecurity professionals could leverage generative AI to anticipate threats before they materialize, and to maximize the value of their existing security tools. Generative AI enables enterprises to take a proactive approach to cybersecurity.
Generative AI can also be instrumental in helping teams secure their systems. For instance, it can be used to generate complex, unique passwords or encryption keys that would be extremely difficult to guess or crack. Because weak or compromised credentials often serve as entry points for security breaches, generative AI can offer an additional layer of security.
Pros and cons of generative AI in cybersecurity
Generative AI in cybersecurity brings significant advantages, offering solutions to many of the challenges faced by cybersecurity professionals today.
- Efficiency: With generative AI, cyber threat detection and response can become more efficient. As an AI-native system learns how to perform certain tasks, it can help security analysts surface information that they need to make decisions quickly. This accelerates analyst workflows, freeing them to focus on additional tasks, thereby scaling their team’s output.
- In-depth analysis and summarization: Generative AI can enable teams to analyze data from different sources or modules, enabling teams to conduct traditionally time-intensive, tedious data analysis with speed and precision Generative AI can also be used to create natural-language summaries of incidents and threat assessments, further accelerating and multiplying team output.
- Proactive threat detection: Perhaps the most significant advantage of generative AI is the shift from reactive to proactive cybersecurity. By alerting teams to potential threats based on learned patterns, generative AI allows for preemptive actions before a breach occurs.
Although the use of generative AI is compelling, it’s important to consider the challenges that come with it. Like any technology, its use must be approached responsibly to mitigate risks and potential misuse.
- High computational resources: Training generative AI models requires substantial computational power and storage. For smaller organizations, this can be a limiting factor.
- Risk of AI being used by attackers: Generative AI models and related tools are becoming more and more accessible through open-source, inexpensive, and cloud-based means. Just as enterprises can leverage generative AI for cybersecurity, cybercriminals can use generative AI to develop sophisticated attacks that are adept at evading cybersecurity measures. Through a growing ecosystem of GPT-based tools, generative AI is lowering the barrier for new threat actors to conduct highly sophisticated attacks.
- Ethical considerations: Current conversations are raising ethical questions related to privacy and control over data, especially when it comes to the type of data used by AI models in training datasets.
Introducing Charlotte AI: an AI-native security analyst
As a use case of generative AI in cybersecurity, CrowdStrike is one of the first vendors to have introduced a security analyst known as CrowdStrike® Charlotte AI that reduces the complexity of security operations for users of any skill level. As users ask Charlotte AI questions (in dozens of languages), the Charlotte AI engine interacts with the CrowdStrike Falcon® platform and responds with intuitive answers.
Charlotte AI can provide real-time insight into an organization’s security posture, acting as an intelligent security analyst that works for the enterprise. Charlotte AI enhances the capabilities of cybersecurity professionals, helping them make better decisions faster, using the rich, real-time data of the Falcon platform.
Charlotte AI is also purpose-built for security analysts, with sensitive data redaction to ensure user privacy, auditability to ensure accuracy and minimize AI hallucinations, and built-in role-based access control, to ensure that data is surfaced only to authorized users.
We expect to see many more positive AI enhanced security agents like Charlotte operating off other vendors’ large data models in the next few months which we believe will begin to advance our ability to leverage GAI for the common good and we are looking forward to it.
Author
Steve King
Managing Director, CyberEd
King, an experienced cybersecurity professional, has served in senior leadership roles in technology development for the past 20 years. He has founded nine startups, including Endymion Systems and seeCommerce. He has held leadership roles in marketing and product development, operating as CEO, CTO and CISO for several startups, including Netswitch Technology Management. He also served as CIO for Memorex and was the co-founder of the Cambridge Systems Group.