blog post
Adapting to the Unpredictable: The New Cyber Frontier
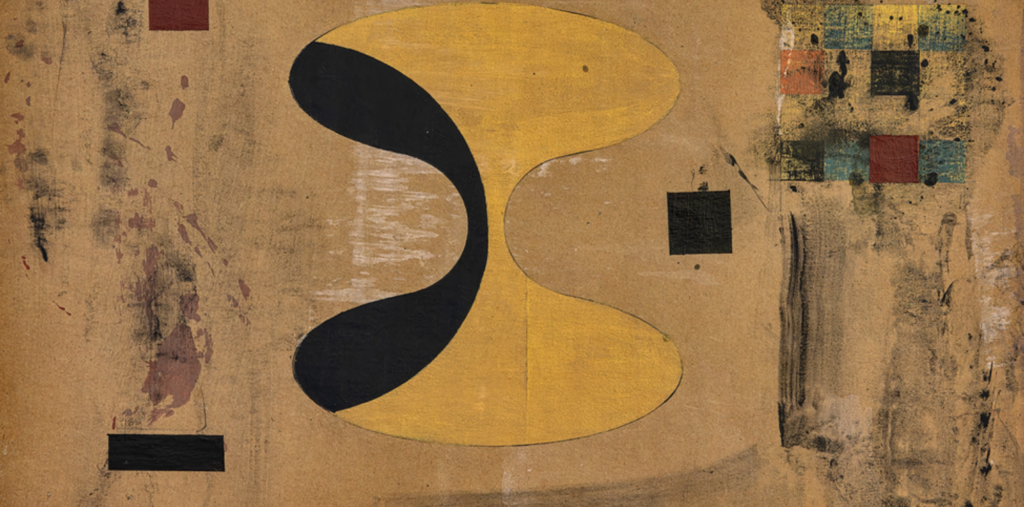
When artificial intelligence arms cybercriminals with the tools to craft highly targeted and unprecedentedly unique attacks on a massive scale, the battleground of cyber defense seems locked in a perennial game of catch-up. The traditional arsenal, heavily reliant on the archives of historical cyber skirmishes to predict and parry incoming threats, finds itself outpaced in a landscape where the unconventional becomes the standard.
The evidence of this shift is stark: a staggering 135% surge in sophisticated social engineering tactics coincides with the rapid integration of technologies like ChatGPT from January to February 2023, signaling a paradigm shift in attack methodologies. Meanwhile, the response time for legacy defense mechanisms to recognize and respond to an email-based assault averages a sluggish 13 days—an eternity in the digital realm.
Moreover, the period between May and July 2023 witnessed a 52% climb in the hijacking of email accounts, underscoring the pressing need for a revolution in our defensive strategies. As the digital adversaries evolve, so too must our defenses, lest we find ourselves perennially far behind in the ceaseless cyber war.
A Significant Shift
This rise of AI in orchestrating cyber-attacks marks a significant shift, with tools such as generative AI and large language models (LLMs) equipping even inexperienced attackers with sophisticated offensive capabilities. This evolution has necessitated a parallel advancement within the cybersecurity sector, prompting the integration of AI-driven defenses aimed at enhancing prevention, detection, response, and recovery measures. This strategic deployment is essential for keeping pace with the rapidly changing cyber threat landscape.
One Size Does Not Fit All
However, the effectiveness of AI in cybersecurity is not uniform across all implementations. Various AI systems offer distinct advantages and limitations depending on the cybersecurity challenges they address.
The majority of current security technologies lean on AI models trained with datasets of known attacks. This approach, while useful, inherently restricts the system’s ability to identify only those threats that mirror or closely resemble previously encountered attacks. As cyber adversaries continuously innovate, launching unprecedented attacks at an alarming rate and magnitude, the reliance solely on this supervised learning model falls short.
To bridge this gap, there is an urgent need for AI mechanisms capable of detecting and mitigating previously unseen threats, underscoring the necessity for a more adaptable and forward-thinking AI strategy in cybersecurity.
Using One’s Own Data
Historically, cyber-attacks were somewhat generic, with perpetrators deploying well-known methods and tools against a broad spectrum of targets. However, the advent of advanced offensive AI technologies has paved the way for highly personalized “one of one” attacks. These bespoke attacks are meticulously crafted to exploit the unique vulnerabilities of a specific target, rendering traditional defense mechanisms less effective.
For instance, attackers are now leveraging AI to develop custom malware that zeroes in on the specific weak points of a victim’s security setup. Additionally, AI enables the automation of attacks, enhancing their speed and efficiency. Consequently, the future of cyber-attacks promises to be marked by a heightened level of sophistication and complexity.
While the integration of AI into cyber-attacks is still emerging, its trajectory is clear—AI-driven attacks are set to become more prevalent and formidable.
Evolving Cybersecurity with AI
Traditionally, cybersecurity strategies have revolved around collecting data from numerous sources, analyzing it in massive cloud-based databases to identify patterns of attacks, and then applying those insights to thwart future threats. However, this method falls short when facing new, highly customized attacks.
The new paradigm of cybersecurity, dubbed “one on one security,” emphasizes learning from an organization’s unique data footprint to fend off all manner of threats, including those previously unseen.
Organizations are thus compelled to shift away from the outdated perimeter-based security models, which rely heavily on predefined rules and signatures to block known malicious activities. Even with AI automation, this retrospective approach is inherently limited, constantly lagging behind the evolving threat landscape.
In contrast, self-learning AI systems redefine security norms by continuously analyzing every interaction within an organization’s network—across devices and users—to establish a baseline of “normal” behavior. This deep understanding of an organization’s “self” enables the AI to detect subtle anomalies that could signal an impending attack, offering a dynamic defense mechanism that evolves in tandem with emerging threats.
Such an approach ensures that organizations can not only keep pace with but also proactively anticipate and neutralize novel and sophisticated cyber-attacks, marking a significant leap forward in cybersecurity strategy.
Understanding AI in Cybersecurity: From Supervised Learning to Self-Learning Systems
In today’s cybersecurity landscape, supervised machine learning models stand as a cornerstone, built upon vast datasets of known cyber threats.
These models, pivotal in solutions like Extended Detection and Response (XDR), excel in identifying and mitigating previously encountered cyberattacks through their training on structured, labeled data. Their proficiency forms an essential foundation for any cybersecurity framework.
Yet, their effectiveness is challenged by novel threats, revealing a critical limitation: unfamiliar patterns can slip through undetected, and legitimate data mingled with anomalies can compromise their accuracy. Continuous testing and validation remain crucial to maintaining their reliability.
Contextual Understanding at Scale
Trained on extensive internet data, LLMs are revolutionizing human and machine language applications, promising significant advancements in productivity and creative processes. However, the integration of these technologies into the workplace raises concerns over privacy, potential data leaks, and the inadvertent sharing of sensitive company information. In the realm of cybersecurity, generative AI offers the advantage of large-scale contextual understanding and the automation of tasks like incident reporting.
Yet, the risk of generating inaccurate or misleading information, alongside the inherent vulnerabilities to prompt injection attacks, calls for cautious and responsible application.
Self-Learning AI represents an advanced, multi-faceted approach in artificial intelligence, combining numerous techniques and models to adapt and respond to the unique digital ecosystem of each organization.
Unlike their predecessors, today’s Self-Learning AI doesn’t rely on historical attack data but instead learns directly from the organization’s ongoing activity. It’s designed to discern what’s normal within a specific context and detect anomalies in real-time, enabling it to counteract not just known threats but also emerging challenges such as zero-day exploits, insider attacks, and sophisticated phishing operations powered by AI.
Foundational Principles
Modern Self-Learning AI models are built on four foundational architectural principles: it must learn continuously from the organization’s live data environment, it must thrive on the complexity inherent in modern enterprises, it must dynamically adjusts its understanding of normal behavior through probabilistic analysis, and it must operate autonomously, without the need for human oversight.
This approach ensures that the AI remains ever-evolving, capable of defending against the latest cyber threats in an increasingly complex digital world.
Author
Steve King
Managing Director, CyberEd
King, an experienced cybersecurity professional, has served in senior leadership roles in technology development for the past 20 years. He has founded nine startups, including Endymion Systems and seeCommerce. He has held leadership roles in marketing and product development, operating as CEO, CTO and CISO for several startups, including Netswitch Technology Management. He also served as CIO for Memorex and was the co-founder of the Cambridge Systems Group.