blog post
AI in Discussion
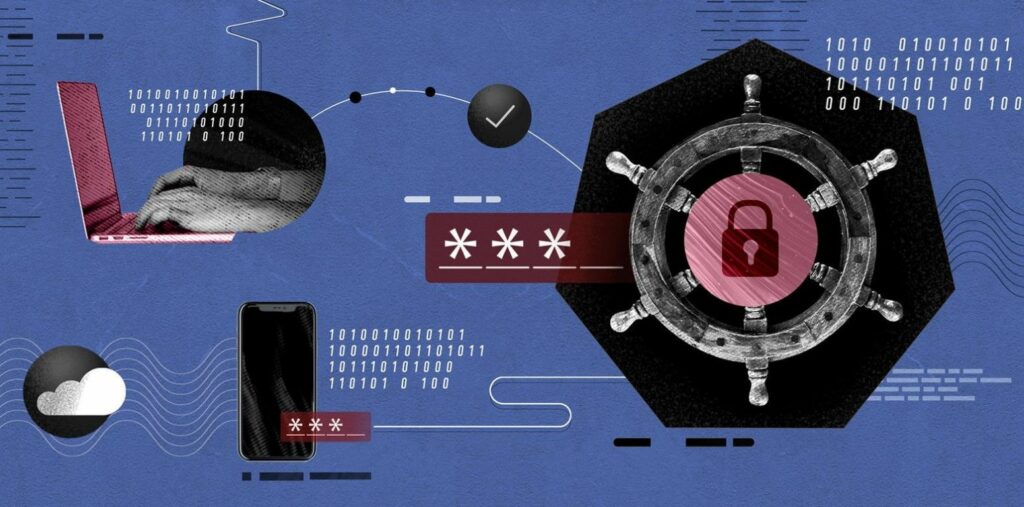
During the week of July 10th, Fortune held its 22nd annual Brainstorm Tech conference in Park City, Utah.
Speakers ranged from former VP Al Gore, the creator of the Internet to athletes Lindsey Vonn, formerly of Tiger Woods fame and Andre Iguodala, the GSW oft-injured swingman, né newly minted venture capitalist to WeWork founder, billionaire and preacher, Adam Neumann.
Vishal Shah, Meta’s VP of Metaverse; Arati Prabhakar, director of the White House Office of Science and Technology Policy; and Bryan Johnson, the Braintree founder who is still seeking eternal youth, all participated on 3 panels.
A.I. was front and center in each discussion. For Shah, A.I. is foundational to his work on the metaverse—he can’t build it or lure creators onto the platform without continued advancements there, implying that maybe the metaverse isn’t quite as dead as many had hoped.
Johnson feels we are on the cusp of “superhuman intelligence” thanks to A.I. He predicts it will enable us to create genius-level inventions much more frequently than we have in the past. Competing with other genius-level stuff like spreading misinformation and propaganda on a massive scale, wreaking havoc on critical infrastructure and financial systems, and creating autonomous weapons that can make their own targeting decisions based on social credit scores.
Prabhakar is focused on mitigating the risks of A.I. by creating future-proof guidelines and values. She also said it’s a “global race to get A.I. right,” and that multinational agreements with like-minded nations are critical.
While we cast about for like-minded nations, it might be a really good idea to get our minds wrapped around AI, and its place in the cyber-universe. Many folks think of AI in the G-AI context and imagine lots of automation opportunities in SAC, Operations, SOAR, DevSecOps, etc.
But I’m sure many are not thinking about the downsides and risks associated with all of the use cases that pop up in those contexts. We can get G-AI to write code for us, but it might just make up some stuff that doesn’t exist, which can then be replaced with malware once the new code is in the repo.
G-AI is really good at creating stuff and relies upon a corpus of ‘knowledge’ birthed in the Internet to do so. And its knowledge models will contain some good stuff and other bad stuff. It has no way to determine the difference, so we get what we get.
Discriminative AI, on the other hand is single-purposed, been around a long time and used for supervised machine learning. They do what they ‘literally’ say, separating the data points into different classes and learning the boundaries using probability estimates and maximum likelihoods.
More time spent on discriminative and less time spent on generative might yield better results in cybersecurity.
Author
Steve King
Managing Director, CyberEd
King, an experienced cybersecurity professional, has served in senior leadership roles in technology development for the past 20 years. He has founded nine startups, including Endymion Systems and seeCommerce. He has held leadership roles in marketing and product development, operating as CEO, CTO and CISO for several startups, including Netswitch Technology Management. He also served as CIO for Memorex and was the co-founder of the Cambridge Systems Group.